One data layer is never enough in precision ag
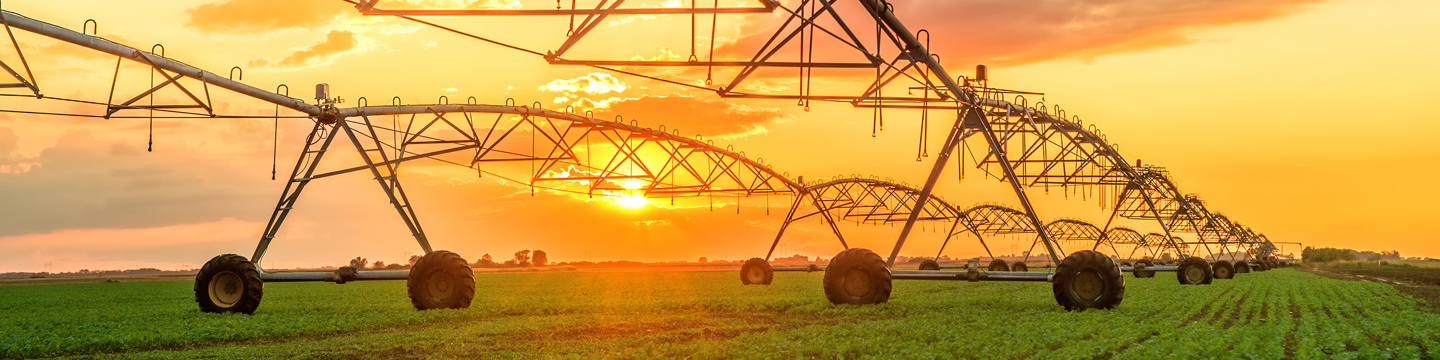
By Jason Tatge, Farmobile co-founder and CEO
. . .
Precision agriculture is in a unique moment – we’ve been talking about the promises of the technology for 30+ years, but we’ve yet to realize it’s full potential. The most fundamental issue remains the same – without an accurate underlying data set, we can be precise only to a degree. Now, however, with new technologies coming online we have more opportunity than ever to get more precise intelligence about the way we manage the rich, ground-truthed data that’s all around us.
“What if we were able to pair that (satellite imagery, weather stations, soil moisture sensor, etc.) data with a foundational layer of accurate, ground-truthed data, that augments and validates these other sources with a new sense of precision – in real-time?”
We already have plenty of rich data sources in agriculture – like satellite images, weather stations and soil-moisture sensors – that have given us more insight than ever before. But, what if we were able to pair that data with a foundational layer of accurate, ground-truthed data that augments and validates these other sources with a new sense of precision – in real-time?
Precision ag is a shortcut
My friend Steve Cubbage calls the process of collecting data directly from the field “real” agronomy. And I have to agree with him. Today, going out into the field and holding the soil in your hands, grabbing a tissue sample, counting bean pods and measuring the circumference and length of an ear of corn is the best thing we’ve got to predict yield and diagnose and treat the problems.
But walking out into the field for every insight is hard. And, it isn’t exactly scalable or consistent across workers, which is why the promise of precision agriculture is so alluring. Imagine software that promises to leverage the predictive power of Artificial Intelligence to tell farmers and ag retailers exactly how much fertilizer to lay down, exactly how many seeds to plant per acre, exactly which varieties and fields give you the highest probability of a positive ROI on a fungicide application – and make these fields more profitable than ever before? Sign me up!
Now, we’re starting to collect an even richer and more precise set of data – a baseline of raw, machine-generated, field-level data from which Machine Learning (ML) systems can begin to “learn”. And the basic premise of all ML is that it’s only as good as the data it gathers. In agriculture, we need a lot of ML data before we can move to Deep Learning and then to Artificial Intelligence.
To be clear, all forms of data currently being fed into precision systems are not bad. In fact, they can be a very useful pieces of the “information pie”; but without real, ground-truthed, geo-referenced points in the field, it is extremely difficult to correlate actions to results and impossible to replicate with any sort of consistency.
Think of today’s weather data
It is fair to say that weather data is ubiquitous and – with the tap of an app on your phone – instantly available via a combination of satellite imagery and radar. It’s often not perfect, but it’s decent. But what really starts to drive meaningful differences in the quality of weather data and turns it into weather information is when it is combined with close-proximity, ground-based weather stations that update at a high reporting frequency. These ground-based “weather stations” bring into focus the many layers making all of the data sources more valuable together than individually.
On the farm, the same is true for collecting high quality ground-truthed field-level data. If we rely only on the external ubiquitous data available today, we are making “best guesses” as to what may be going on in the field. These “best guesses” without the addition of the ground-truthed field level data is a major reason precision ag has historically underperformed from an ROI perspective. But with the combination of ground-truthed field level data and external ubiquitous data layers, we can start to make the shift from correlation to causation, and a new level of understanding about what’s really happening in the fields is on the horizon.
New heights for satellite data
Let’s use satellite imagery as an example, since it’s been in the ag news quite a bit in the last couple of months (here and here).
Aerial imagery isn’t new. It’s more readily available today than ever before thanks to advances in satellite technology and affordability. It’s good for telling you the temperature of a crop and how much vegetation is growing; but it’s not very good, yet, at telling you how much yield potential you have. And, it’s certainly not good at tracking actual results at the end of the harvest. That’s not what it’s built for.
And yet, it was surprising to me to find out how heavily the industry (specifically ag tech firms which attempt to replace boots-on-the-ground) relies on satellite data for writing fertility prescriptions, which if done properly, require the understanding of how much crop the soil actually produced last year (yield) to make the proper, environmentally aware, fertility recommendations for next year. Predicted yield is the exact thing that today’s satellite data is not very good at. As a commodity trader, we’d never make our bets on satellite data alone. We needed some ground-truthed data to verify what indications we interpret from the satellite data.
Here’s an example: Nitrogen accounts for 30 percent of a corn crop’s yield. Most agronomy firms today use satellite imagery to estimate the actual yield on that particular field. From that “best guess”, they create a prescription that suggests where the fertilizer should be applied and what rates should be used. Many times, the story on the ground is vastly different. Competitive ag tech firms generate prescription maps from satellite images that are never actually calibrated with reality – and the variation is often not a little bit, but a lot. Because most farmers are hiring custom applicators to apply many of their fertility layers, it is often difficult to track the difference between what was prescribed versus what was actually applied.
In general, the industry follows a ‘better too much, than too little’ credo, even when less might actually be more fruitful and more cost-efficient. To be clear, up to today, this is no one party’s fault. It has been the great disconnect that divides this industry, but we now know how to fix it.
Nothing is as good or effective as collecting real ground-truthed data from the machines and augmenting this with “real” agronomy.
Conclusion: ground-truthed data is key
Ag retailers have taken this industry to incredible lengths working with tools that have been traditionally available to them. As we move to the next chapter, we now have low-cost digital tools to accurately collect, measure and share data, that will help farmers realize productivity and profitability gains never before possible. Also, I believe we will see a new level of transparency as these ground-truthed data layers all come together.
The real opportunity exists in agriculture to update our incentive structures in such a way that ag retailers benefit from sharing how their practices of applying the right amount of fertilizer in the right places on the fields at the right time become the real differentiators. I believe this will come from a combination of expanded “real” agronomy practices combined with precision ag experts, who will deliver results like we’ve never seen before. Aligning incentives structures based on outcomes rather than the total amount of tons applied will change this industry forever.
Innovative applicating services, that combine past and forecasted weather, soil samples, previous crops, tillage practices, and actual ground-truthed planting and harvest data, is the service offering of the future.
- Farmers will benefit with better-performing crops.
- Ag retailers will benefit from a better understanding of actual results.
- And, the whole industry would be accountable and transparent to itself and to consumers, who buy agriculture’s products and, ultimately, affect production practices and subsequent regulatory policy.
This is all a pipe dream unless we have a foundational layer of ground-truthed, machine-generated data collected from the field. Agriculture is a hard industry, and there are no easy shortcuts. Yesterday’s tools will not be enough to stay in business tomorrow. I can’t imagine a better time to be sitting at the intersection of agriculture and technology!
This is why Farmobile has invested so much in the heavy lifting of creating the world’s largest digital library of ground-truthed, science-worthy, farmer-certified data. And, we’ve made it available for sharing and licensing. It will be this foundational platform – performing the hard work of collecting, processing and organizing these new geospatial digital assets – that unlocks the first generation of true Machine Learning in agriculture.
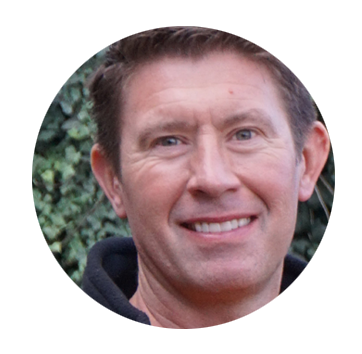
Jason Tatge
Farmobile co-founder and CEO. Passionate about advocating for the farmer. #FarmerPower.